Welfit Oddy’s Seamless Success
Optimising Welding Efficiency

IMPACT
- More accurate picture of machine usage
- Better asset care
- Enhanced productivity
- More accurate costing for customers
year old machine
Raven sensor
key dashboards
The Customer Journey
About The Customer
For over a century, Welfit Oddy has been a trailblazer in transport equipment manufacturing. The company pioneered the intermodal tank container market in the mid-1980s and today remains a global leader in the design and production of these tank containers. Every day, its Gqeberha facility produces 30 of these versatile units, which are used for transporting liquids, gases and bulk materials across ships, trains and trucks.
Welfit Oddy has earned its reputation for creating innovative and efficient tank container solutions that cater to diverse transportation requirements.

Beyond Automation
Automation has become a cornerstone for enhancing productivity and quality in modern manufacturing. For example, automated linear welding machines have revolutionised the welding process by streamlining the creation of precise metal joints. While these machines have become indispensable tools and significantly improved efficiency, there are still challenges in optimising their operation to eliminate bottlenecks and ensure consistent output.
Key Challenges
Despite their advantages, Welfit Oddy’s linear welders often encountered inefficiencies, including extended idle periods and inconsistent welding times, which resulted in reduced productivity, increased labour costs and compromised weld quality.
Being able to track when a machine was in use or idle, and what was happening during that downtime, was a critical need for Welfit Oddy in order to provide more accurate costings for customers.
Having real-time insights into the welding process helped to highlight irregularities that might result in a breakdown, which enabled them to act before problems occurred and ensured more efficient use of the linear welders.
Integrating the Raven sensor into the ODIN Checkpoint dashboard offers the capability to delve deeper into operational patterns.
Digital Solution 1
Real-time Insights and Predictive Analysis
The Raven sensor, a cutting-edge, pocket-sized piece of hardware, integrated with the ODIN Checkpoint platform, was introduced to monitor and optimise the operation of working equipment – sampling the temperature and vibration to predict the operational state of the asset/machine. This sensor provides real-time data on welding times, enabling immediate intervention to rectify production issues. By ensuring consistent and predictable times, the sensor contributes to a reduction in labour costs and an enhancement of overall productivity.
Moreover, the sensor’s integration with machine learning at the edge enables the prediction of current welding operations and tracks the duration of welding activities.
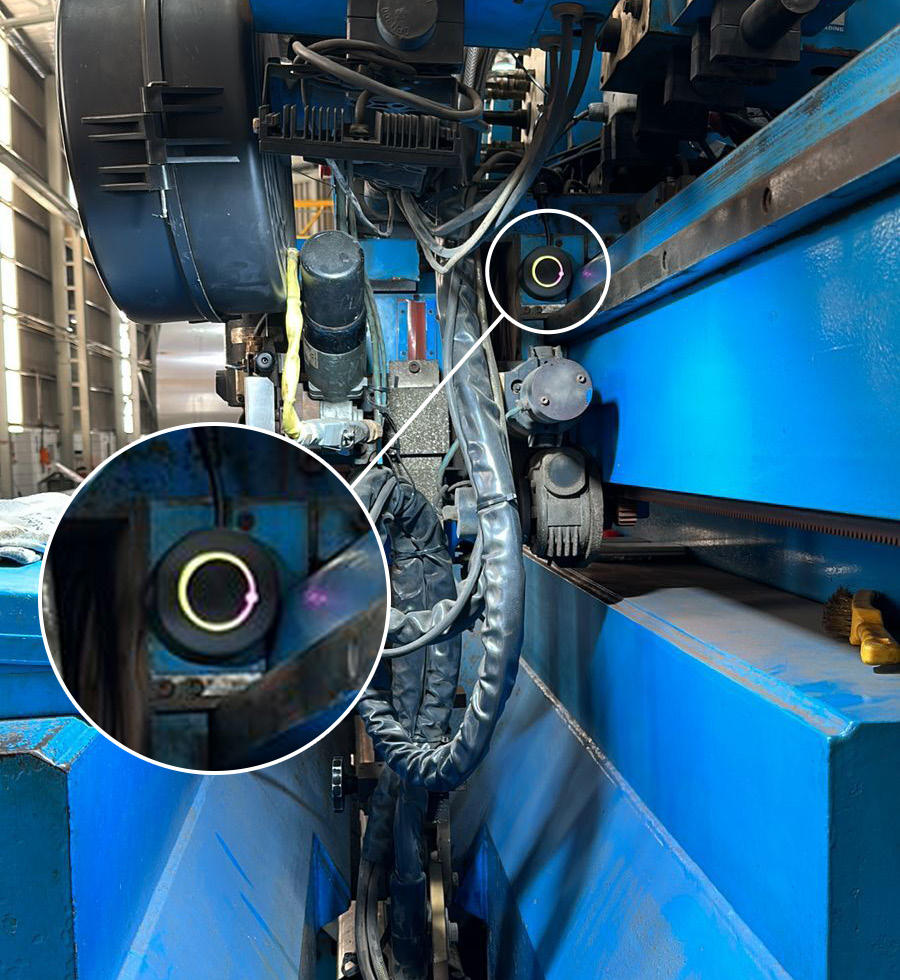
Digital Solution 2
Enhanced Visibility and Informed Decision-Making
ODIN Checkpoint offers a suite of interactive dashboards that empower users to gain valuable insights into the operation of linear welders.
Users can:
Get a holistic view of production performance:
Monitor the overall usage of the welding machines over defined periods e.g. the total number of welds conducted during each hour of a day. This feature helps to determine whether production targets are being met and identify periods of inefficiency, such as prolonged idle times or loading activities.
Access accurate info for calculating part production costs:
The dashboards facilitate a detailed analysis of welding costs by estimating the expenses associated with individual welds. This estimation is based on the average time required to complete a weld.
Additionally, users can derive insights into the number of welds needed to manufacture specific parts, thereby contributing to informed decision-making and cost-effective planning.

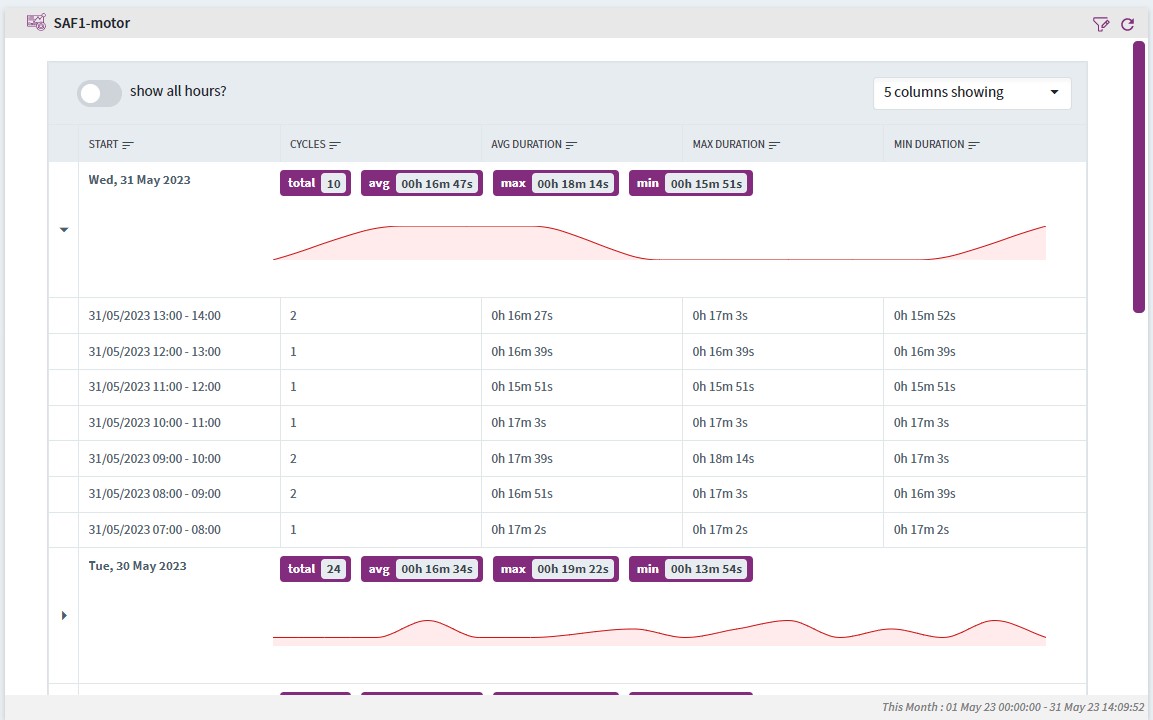
Deep dive analysis
Uncovering Operational Patterns
Integrating the Raven sensor into the ODIN Checkpoint dashboard offers the capability to delve deeper into operational patterns.
By drilling down into usage data for specific shifts, days, or weeks, users can pinpoint productive and unproductive periods.
This granularity of analysis enables management to identify and address extended periods of inactivity during shifts.
By collaborating with operators to mitigate these unproductive intervals, management can further optimise the overall efficiency of the welding process.
Conclusion
The successful implementation of the Raven sensor and ODIN Checkpoint platform demonstrated how real-time data monitoring and predictive analytics can enhance the performance of automated welding processes.
By providing real-time insights, predictive analytics, and comprehensive dashboards, this solution enables businesses to overcome challenges related to inconsistent welding times, prolonged idle periods and sub-optimal resource utilisation.
As a result, industries relying on linear welding machines can achieve heightened productivity, reduced labour costs and improved weld quality.
The synergy between cutting-edge technology and data-driven decision-making has ushered in a new era of efficiency and competitiveness in the realm of automated welding.